A Tale of Two Images
If you were asked which of these two images ChatGPT generated when I gave the simple prompt, “create an image of a successful businessperson", which would you guess?
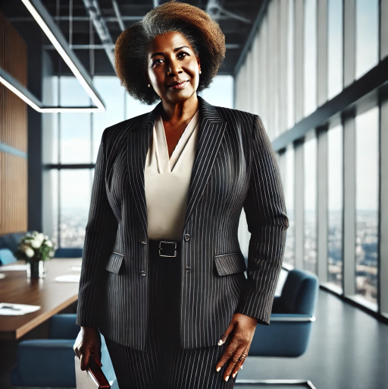
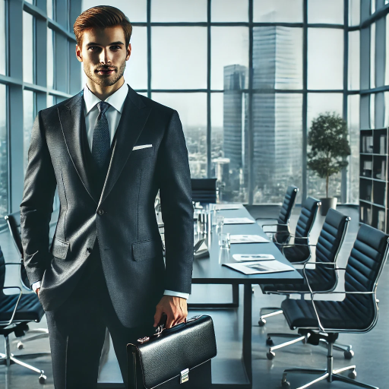
If you are aware of the potential biases in generative AI, I'm sure you've worked out that it's the image of the businessman. Without any further instructions, the AI defaulted to producing an image of a young, white, slim man. But, when I prompted more specifically - for a fuller-bodied black woman in her 50s - it generated an image that just as accurately represents a successful businessperson.
The truth is, generative AIs such as ChatGPT, Copilot and Gemini often reflect the biases embedded in the data they are trained on. Left to their own devices, they can reinforce societal norms and stereotypes. But when we’re more intentional with our prompts, we can create outputs that are more representative and inclusive of the diversity we see in the real world.
Why Does This Matter in Speech and Language Therapy?
Good question. The same underlying biases that affect something as simple as an image prompt can have a much bigger impact when AI tools are used in healthcare. As speech and language therapists, our clients come from diverse backgrounds, with unique needs, cultures, and communication styles. If the AI tools we use are biased, the materials and resources we create could unintentionally exclude or misrepresent the very people we’re aiming to support.

This isn’t just theoretical. AI bias is already affecting healthcare in significant ways. For example, during the COVID-19 pandemic, AI models that were supposed to predict healthcare outcomes turned out to be less accurate for minority ethnic groups. Why? Because the data used to train those models wasn’t representative (Imperial College London). That’s a serious problem when AI is involved in decisions about who gets care and how much.
Example: Bias in a Social Story
Let’s look more specifically at speech and language therapy. Imagine you’re using an AI tool to generate a social story for an autistic child about starting school. You type in a simple prompt: “create a social story about starting school for an autistic child.” The result? Perhaps a story about a child who uses speech to communicate, sits still and looks when they are listening, and has two parents who help them get ready for their first day.
But here’s the issue: the AI has defaulted to neurotypical assumptions about

communication and a stereotypical family structure. Not only does this fail to resonate with the child it’s intended for, but it also reinforces outdated ideas about what "normal" looks like.
If, instead, you adjust the prompt to say, “create a social story about starting school for a non-speaking, autistic child who uses a communication device, needs sensory tools to aid regulation and lives with their Dad” the AI produces something far more relevant and supportive for the child’s unique situation.
If you further prompt it with, “the story should follow Carol Gray’s criteria, for Social Stories with a higher ratio of descriptive and perspective sentences. It should use simple, short sentences, have a positive and reassuring tone, and be written in the first person”, you will achieve a much better-quality social story overall – your attention to detail in the prompting is the key.
More Visual Examples of AI Bias
The messages we communicate through our prompting can make the difference between a client identifying with our therapy resources and sensing that they are meant for them, or feeling disengaged from the whole process. Here are a few more examples to highlight how easily AI defaults to dominant societal norms when we don’t specifically prompt for diversity:
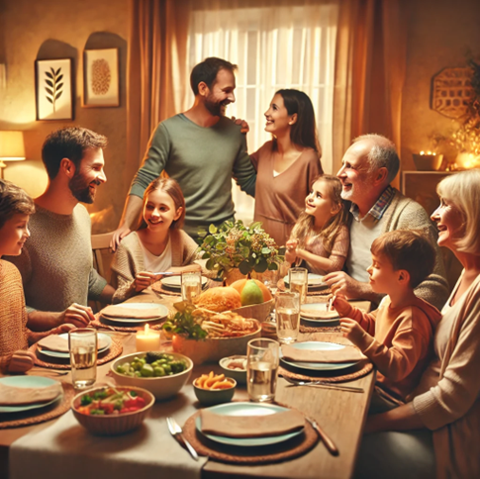
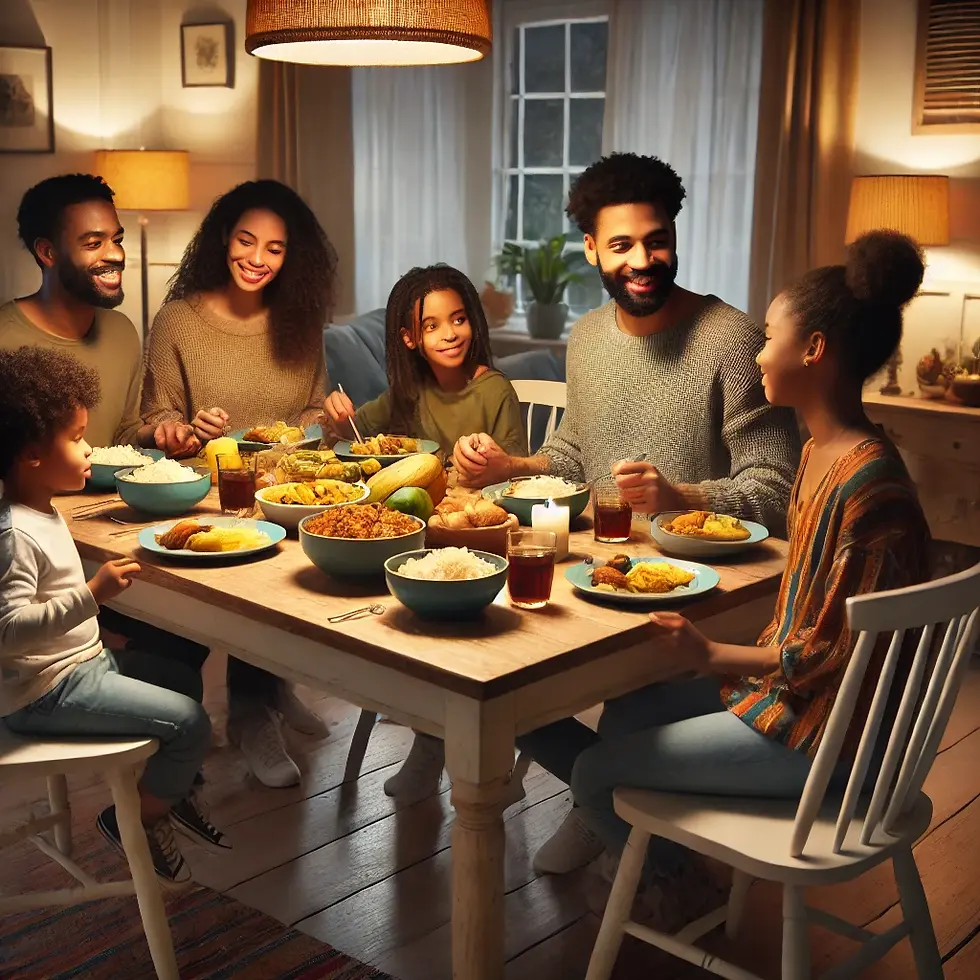
By consciously guiding AI, we can also portray positive, empowering images of disability that align with the aspirations of our service users - challenging outdated perceptions often embedded in AI’s training data.
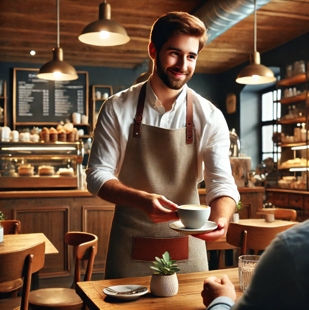
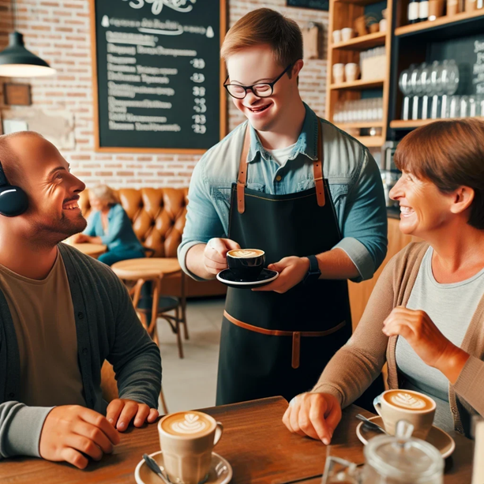
Bias in AI doesn’t just stop at imagery or language - its impact can reach far deeper when used in healthcare decision-making.
Impact of Bias in Healthcare Algorithms
Studies have shown that AI bias can have real-world consequences. For instance, in the U.S., an algorithm designed to allocate healthcare resources was found to prioritise white patients over black patients, despite the fact that black patients had greater medical needs (BMJ). The system relied on biased healthcare spending data, which disproportionately favoured white patients.
The NHS’s work to mitigate such risks is crucial in preventing similar disparities from affecting healthcare delivery in the UK. Fortunately, the NHS is actively working to tackle these issues.
Algorithmic Impact Assessments (AIAs) in the NHS
The NHS is leading the way by piloting Algorithmic Impact Assessments (AIAs), which ensure that any AI system is carefully evaluated for bias before it is rolled out across healthcare systems. AIAs are designed to spot where algorithms might unintentionally perpetuate inequalities, helping ensure that healthcare tools serve everyone fairly, regardless of their age, ethnicity, gender or ability.
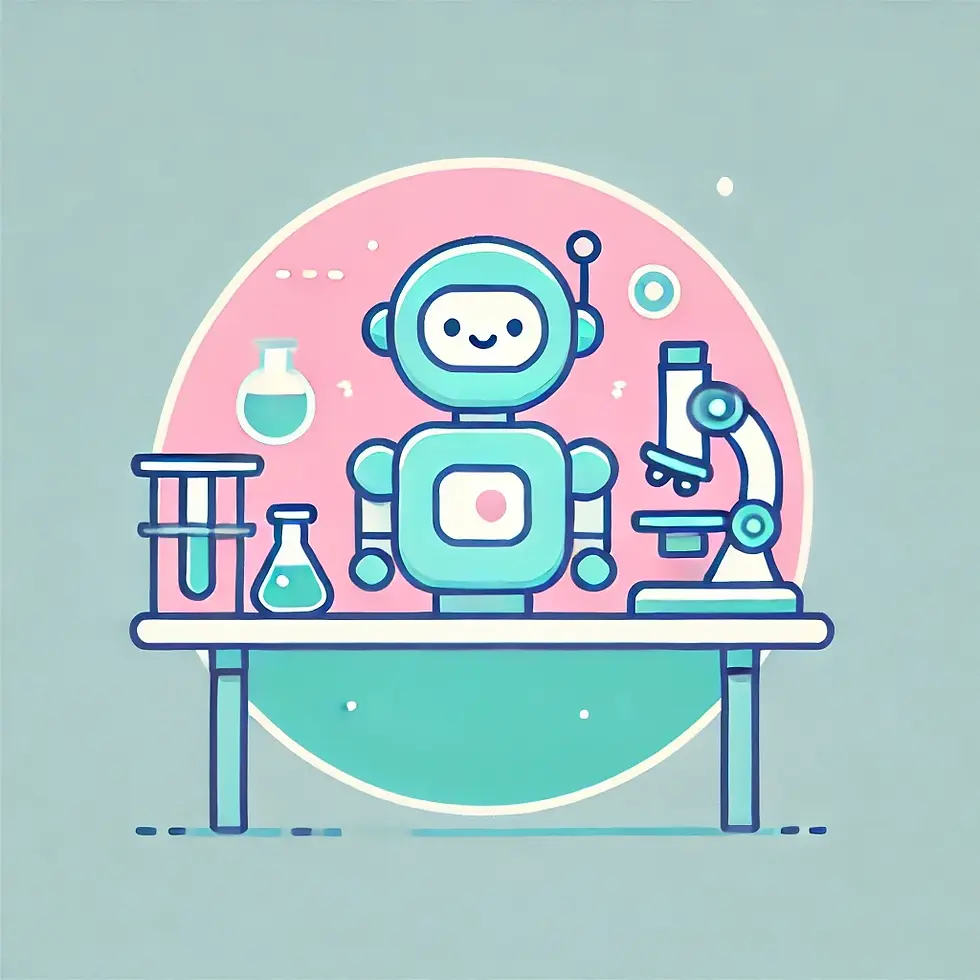
NHS AI Lab’s Ethical Development Initiatives
The NHS AI Lab has been spearheading ethical AI development. The lab focuses on ensuring that AI models used in healthcare are built from datasets that represent the full diversity of the population. It works closely with the Ada Lovelace Institute, which provides tools and guidelines to help developers recognise and mitigate potential biases early in the AI development process.
These initiatives are crucial steps toward ensuring that AI tools, which are becoming increasingly common in healthcare, do not worsen existing inequalities but instead work to close gaps in care.
What Can We Do as SLT Professionals?
While initiatives like the NHS’s AIAs are tackling these issues at a system-wide level, as front-end users of AI tools, we have the power to shape the outputs AI generates. Here are a few practical steps to help you minimise bias in the AI-generated content you use:
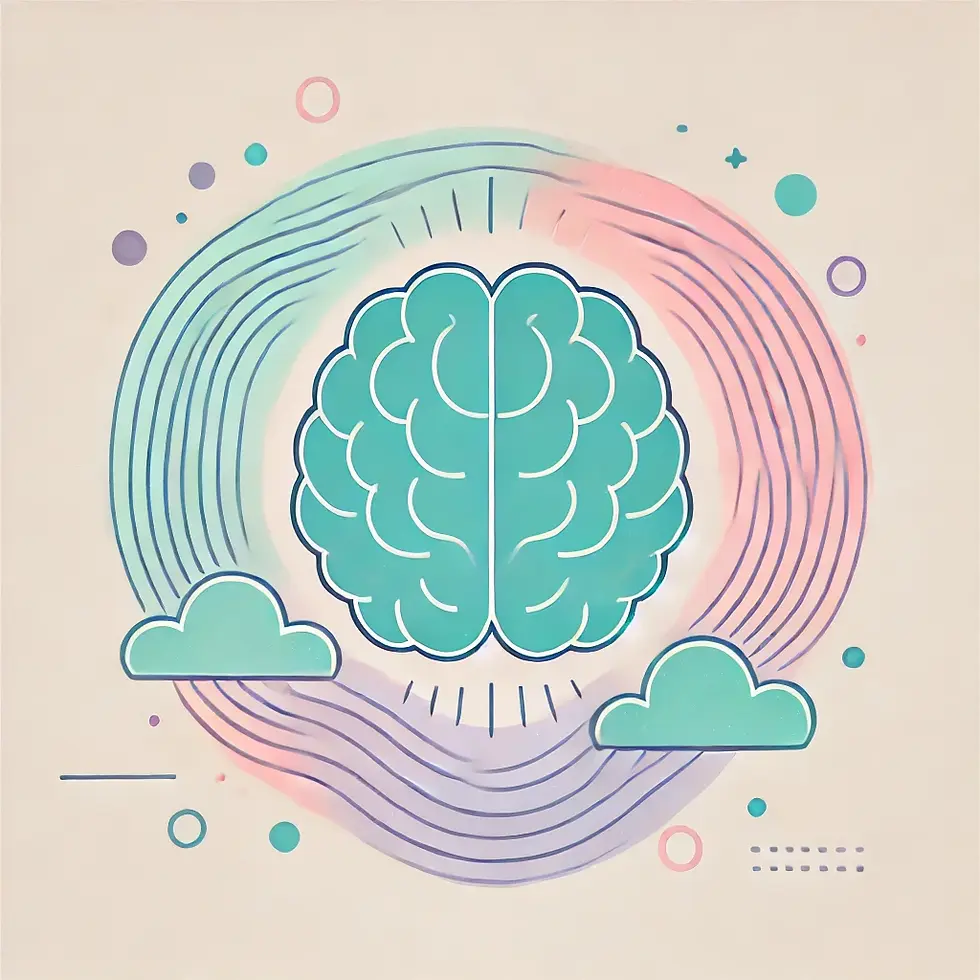
1. Be Mindful of the Prompts You Use
Be specific and intentional with your prompts. For example, instead of asking for: "a story for an 7 year old girl who goes to a birthday party," (which will result in a story that is reminiscent of Enid Blyton’s ‘Famous Five’ books), you might say: "write a story for a 7 year old girl who goes to a birthday party. Do not conform to gender stereotypes, include diversity in culture and ethnicity of the party goers”. This guides the AI away from dominant societal norms leaving you to develop the story to suit your client.
To achieve a more neutral writing style, I recommend using Anthropic's Claude AI which is developed with non-biased, ethical use in mind.
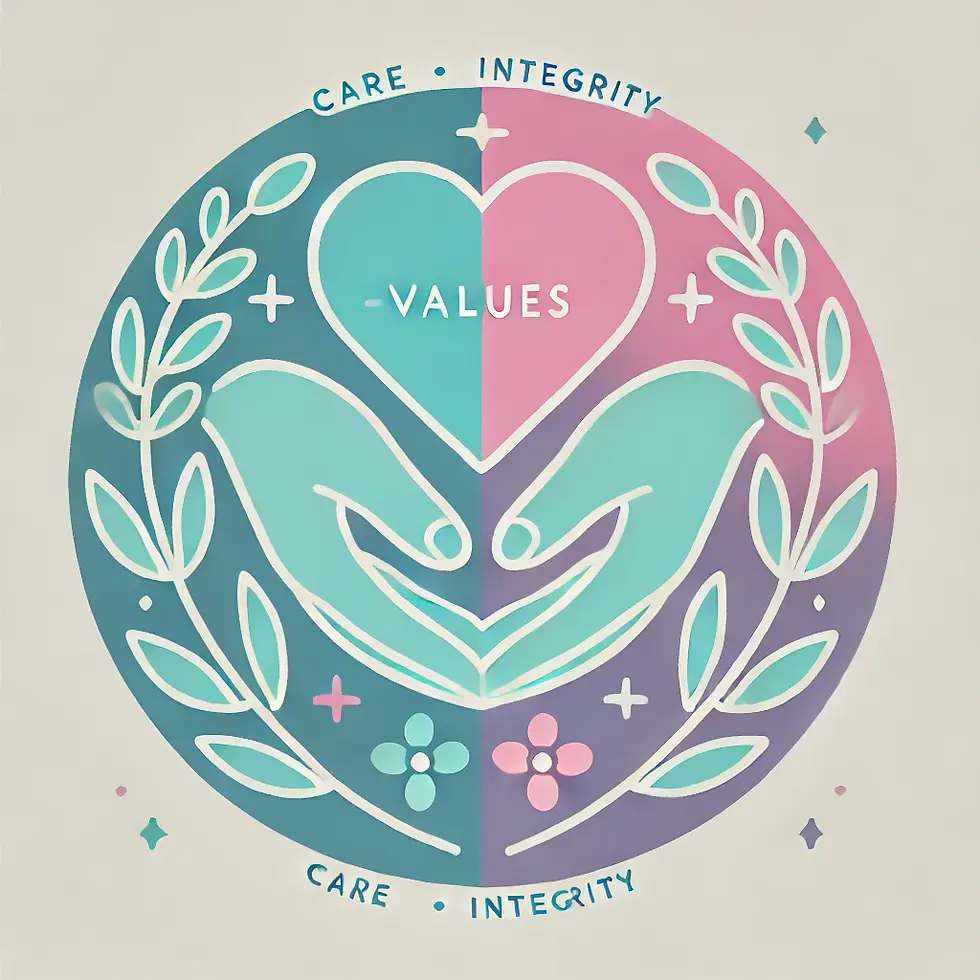
2. Guide AI to Align with Professional Values
It's important to ensure that the language AIs generate aligns with your core professional values. AI can sometimes default to outdated or clinical language, which may not reflect the affirming and inclusive approach you're committed to. For example, within a neurodiversity-affirming paradigm we can prompt the AI to avoid deficit-based language like "suffers with" or "disordered skills," aiming for language which values individual strengths and diverse communication styles. Prompts such as "use strengths-based, inclusive language" or "focus on communication diversity" help guide the AI toward content that supports empowerment and acceptance.
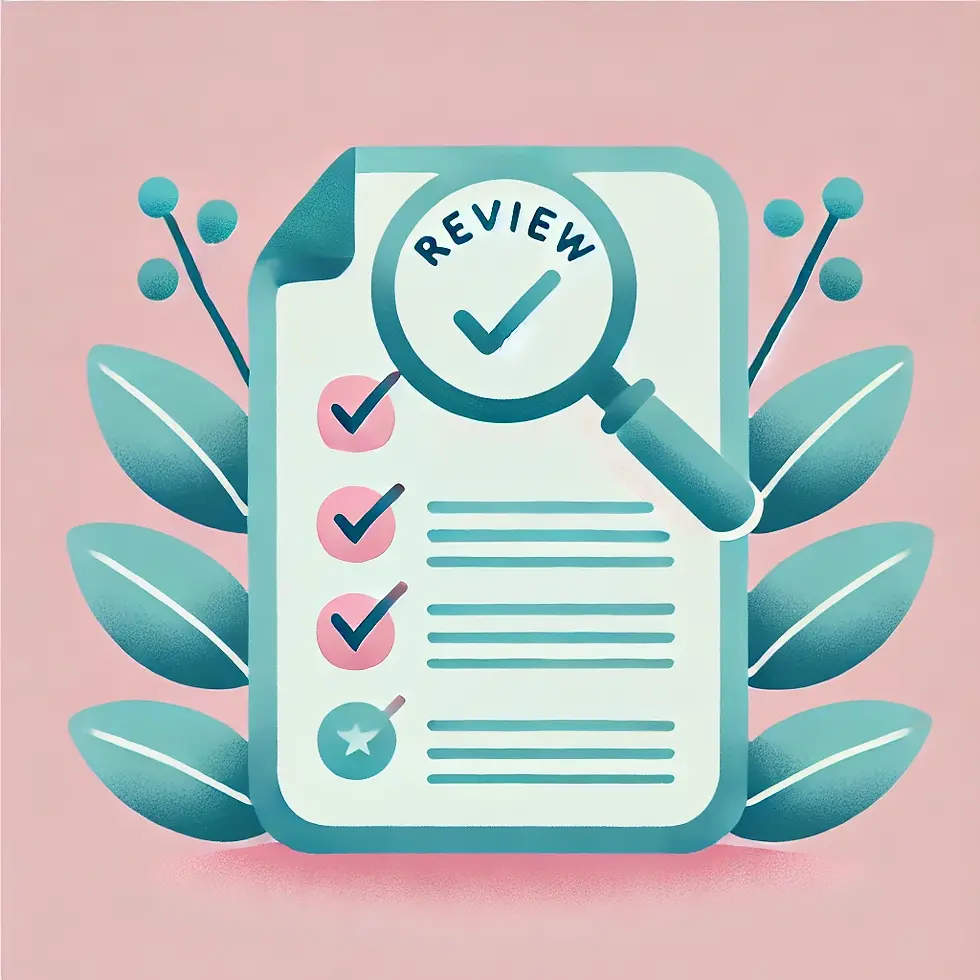
3. Review and Edit AI Outputs for Bias
Even with a well-crafted prompt, An AI might still produce content that reflects bias. It’s important to review the outputs carefully, especially when they involve sensitive topics like culture, ethnicity, gender, sexuality or neurodivergent styles of communication. After generating content, ask yourself: Is the language inclusive? Does the output respect my client’s individuality? If necessary, edit the content to make sure it aligns with best practices in SLT.
For more guidance on crafting effective prompts, check out my blog on the 'SERVE Prompt Framework.' This framework walks you through a clear, step-by-step process for designing prompts that generate accurate, detailed outputs while minimising bias and errors, and ensuring alignment with professional standards
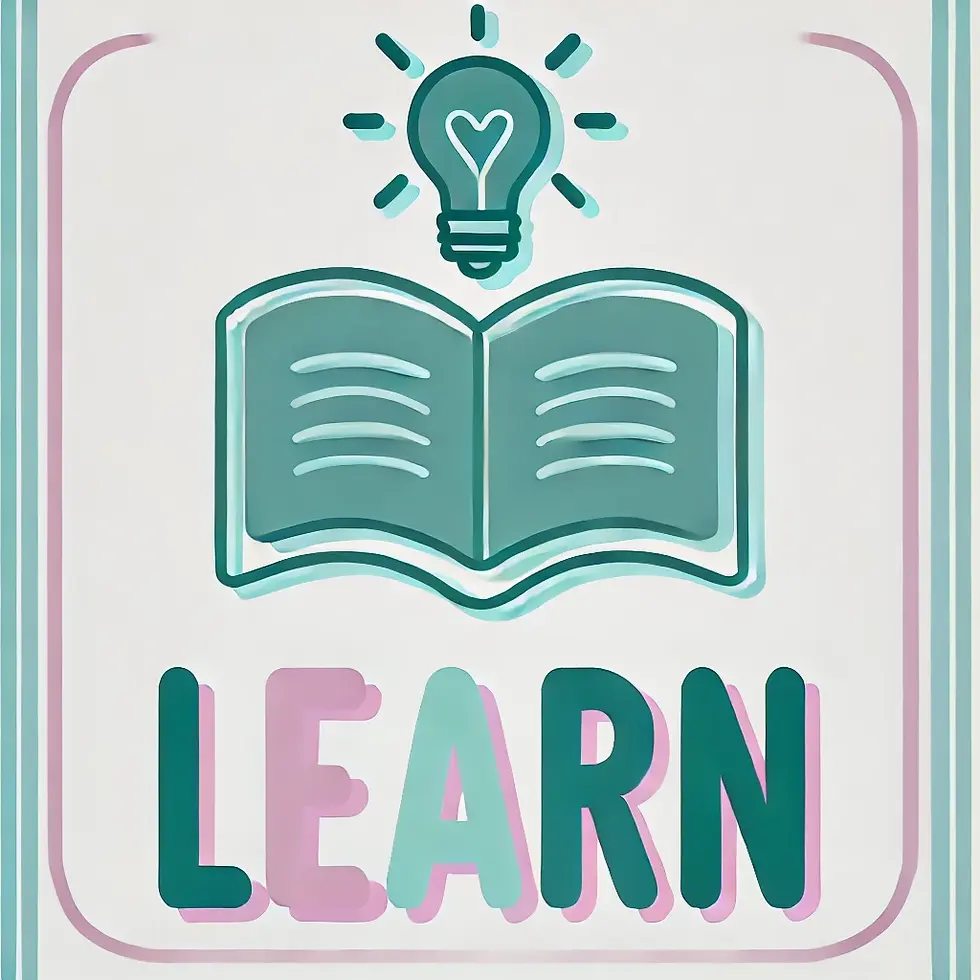
4. Stay Informed About AI and Bias
AI is constantly evolving, and so is our understanding of its strengths and limitations. Stay engaged with webinars, articles, and updates on AI in healthcare. The more informed we are, the better we can advocate for inclusive practices in our work. A list of podcasts, videos, articles and books about AI is available here.

5. Advocate for Better AI Development
As much as we can do as individual users, we also need systemic changes. AI developers must be held accountable for ensuring their models are trained on diverse, representative datasets.
We need to be proactive in providing feedback to AI developers, and advocate for more equitable AI systems.
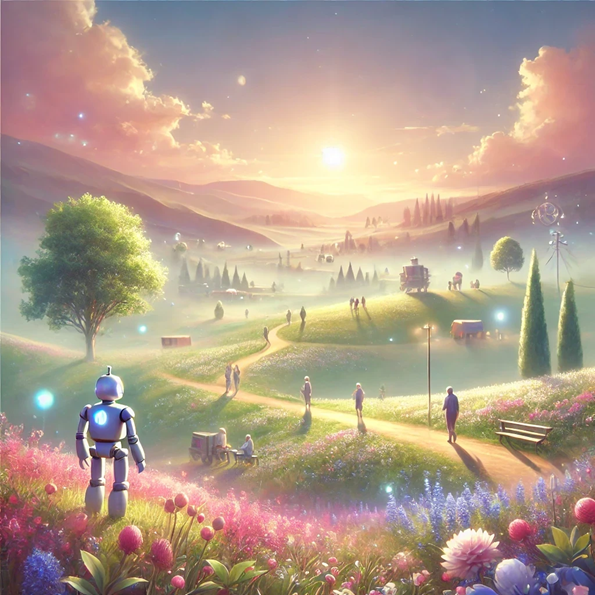
Want to learn more?
Bias in AI is just one of the key topics I cover in my workshops for SLT professionals. In these sessions, we dive into the practical steps of creating effective, bias-free prompts, reviewing AI outputs, and understanding how to use these tools ethically in your practice. If you’re interested in learning more about how to ethically integrate AI into your therapy practice, check out my upcoming workshops, tickets are available here.
Conclusion
AI is a powerful tool, but it’s far from neutral. As speech and language therapists, we carry the responsibility to ensure that AI reflects the diversity and unique needs of our clients. By thoughtfully guiding its use—prompting for inclusivity, reviewing outputs critically, and advocating for ethical development—we can help shape a future where AI supports everyone equitably, without reinforcing the biases of the past.
Note on terminology: This article follows UK convention in using lowercase for all ethnic designations, including 'black' and 'white'. I acknowledge ongoing discussions about capitalisation practices and their implications for addressing bias.
Continue the Conversation:
I'd love to hear about your experiences using AI in SLT. Connect with me via:
AI Acknowledgment:
In the spirit of the topic of this blog, I utilised AI tools including Open AI’s ChatGPT and DALL-E and Anthropic’s Claude in the creation of this content for idea generation, editing, researching, and image generation. All AI-generated content was thoroughly reviewed, edited, and verified by me to ensure accuracy and alignment with my professional expertise.
References & websites
Ada Lovelace Institute. Available at: https://www.adalovelaceinstitute.org/project/algorithmic-impact-assessment-healthcare/
BMJ. Bridging the equity gap towards inclusive artificial intelligence in healthcare diagnostics. BMJ. 2024;384. Available from: https://doi.org/10.1136/bmj.q490
Gray, C. (2014). Social Stories™ 10.2 criteria. Available at: https://carolgraysocialstories.com/
Imperial College London. "AI could worsen health inequities for UK’s minority ethnic groups – new report." Available at: https://www.imperial.ac.uk/news
NHS AI Lab. "NHS AI Lab to tackle racial bias in medical devices." Available at: https://www.nhsx.nhs.uk/ai-lab/
Comments